McAfee specialists have released a report on modern facial recognition systems and how they can be tricked by exploiting some flaws in the machine learning algorithm. In the research, titled “Dopple-ganging up on Facial Recognition System”, experts claim that, by abusing these flaws, the system will confuse people with completely different individuals.
The company developed an advanced facial recognition system, very similar to that used by critical facilities such as airports, border points, among others. The goal of the experiment was to trick the system into confusing Steve (User A) with Jesse (User B), and vice versa.
To do so, the researchers employed a technology known as Adversary Automatic Learning (AML), which aims to deceive models of artificial intelligence by sending misleading inputs. In addition, experts used a GAN (CycleGAN) framework, with the ability to transform one image into a different one.
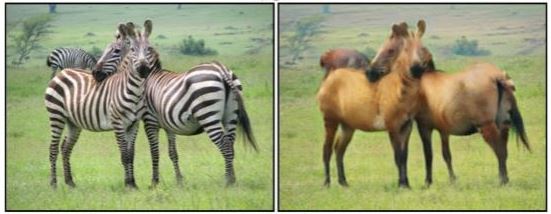
It is worth mentioning that Cycle Generative Adversarial Network (CycleGAN) is a neural network training process for image-to-image translation. CycleGAN is based on modifying some of the most notable characteristics of an image (head shape, eye size, eyebrows, etc.).
When everything was ready, the facial recognition system began to normally detect the faces of users A and B; subsequently, the system trained by the researchers began to combine the facial features of both users, producing candidates that could have passed as valid passport photos. The faces generated by the adversary system seemed so real that in the end it was relatively easy to deceive the target system, which becomes unable to distinguish a computer-generated face.
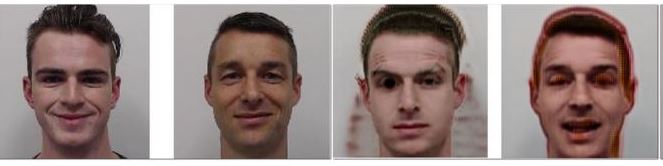
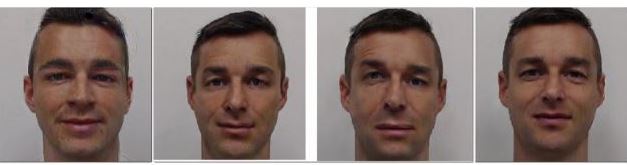
The experiment proved successful for McAfee, which announced that the system misclassified users A and B: “The most important thing is that this method does not compromise the photorealistic appearance of a legitimate user,” the report says.
This is an effort to point out the complete reliance on machine learning systems without considering their potential security flaws. According to the researchers, its main objective is to establish collaboration with the developers of these systems and find the best way to strengthen their security.
He is a cyber security and malware researcher. He studied Computer Science and started working as a cyber security analyst in 2006. He is actively working as an cyber security investigator. He also worked for different security companies. His everyday job includes researching about new cyber security incidents. Also he has deep level of knowledge in enterprise security implementation.